Four Futures
We are in a moment where the future is both wildly exciting and highly uncertain. The acceleration of AI has created a new paradigm of possibilities but also fundamental questions we can’t yet answer. Will we continue speeding towards exponentially growing and continually (potentially self-)improving technology indefinitely or will plateaus, short or long term, slow the train down? Are the upcoming asymptotes near or far away? Will we wind up with a closed ecosystem where single or few rulers reign or an open ecosystem where many models compete and create opportunities on top of them?
The future state and greatest opportunities are likely determined by the two axes central to these questions: rate of advancement and the openness of the frontier models. Rate of advancement indicates how quickly models advance their capabilities towards a self-improving intelligence explosion. Faster advancement means escape velocity is achieved in the next few years, whereas slower advancement would be if one or more plateaus are encountered that delay explosive self-improvement for a decade or more.
Openness is about how much power is held by models and how much profit they can extract as a result. On the open end of the axis, open models are (nearly) as good as closed ones, or alternatively there are many heavily competing closed models of similar quality. In either case on this side models will not be able to capture much of the economics. Closed by contrast means that a few companies with closed models are far ahead and can capture much of the value creation.
While new opportunities will exist across scenarios, we believe the quadrant we land in is likely to dictate what projects, approaches, and types of businesses are most advantaged.

Fat models: lower left quadrant
There has always been a tension and relationship of value between the infrastructure and application layers. In 2016, Joel Monegro, then a USV analyst, published a blog post called Fat Protocols: “the Internet stack, in terms of how value is distributed, is composed of “thin” protocols and “fat” applications. As the market developed, we learned that investing in applications produced high returns whereas investing directly in protocol technologies generally produced low returns.” Instead, in the blockchain stack, this relationship reversed, with more value accruing to the protocols. As AI emerges as a dominant new platform, we again need to figure out this relationship and weighting–does the bulk of value sit with the model or the applications built on top?
In the bottom left quadrant, a world where we continue accelerating the speed of progress in the models and wind up in a closed ecosystem, the models accrue nearly all the value. The more models can do themselves, the less we’ll need apps built on top of them or other models to fill in niches they haven’t covered.
In this scenario, the dominant players will be the small number of giant foundational models and the infrastructure they need to continue to effectively scale. We will need huge quantums of energy (ideally including new forms of more efficient energy.) New opportunities to build and manage this infrastructure–in hardware, software, and energy production–will create massive value. USV portfolio companies like Radiant, Blixt, Lydian, or Glow fall in this quadrant in evolving energy generation and management. There will also be opportunity for counterpositioned model architectures that are making bets on different approaches being the right paths to ultimately winning markets, like decentralized training.
Defensible niche models: top left quadrant
In the top left quadrant, the ecosystem is closed but acceleration slows down, allowing opportunity for niche models to emerge that cover gaps in ability of foundational models. We are seeing quite a bit of this now–biotech, healthcare, robotics, sentiment analysis, personal data sets, physical world data are all examples of opportunities for niche models, some of which we’ve already invested in at USV. Here, data is more specific and targeted than in the generalist models and, often, utility is, too. By shrinking scope and increasing specificity, accuracy and utility can increase. In the world of this quadrant, we continue to see dominant niche models across many categories grow and become important foundations to build on top of.
Network effect applications: upper right quadrant
In the upper right quadrant, the rate of progress in the foundation models slows down and the ecosystem is open. Models have gaps in their abilities and more specific scope, leaving room for startups to productize AI into applications that sit on top.
This outcome is familiar territory for the venture ecosystem. It becomes as much a challenge of imagination as technology. The opportunity is to dream up how to evolve our interactions with AI to change how we work and live. We envision new applications, enablement layers, and interfaces that transform how we learn, research, deliver and receive care, create, play, interact with one another, perform tasks, and manage elements of our businesses. More direct agentic paths to the user, learner, patient, or customer will likely dramatically adjust cost curves and create access. As in previous cycles, many builders will go after the same set of opportunities and so network effects, whether through users or data, will be a critical component in accruing and protecting value.
“Actually open AI”: bottom right quadrant
If models accelerate quickly but within an open ecosystem we wind up with the wildest scenario of all: lots of super smart and rapidly improving models all interacting with each other at high volume and skill. Here, the many open models themselves accrue value as they become dominant in their corners of the market but they all require tooling to interact with one another. In a highly skilled agentic world, agents will need to transact with each other frequently. Crypto may emerge as the easiest and most flexible digital currency for agents, benefitting tokens themselves, decentralized infrastructure, and protocols where agents can interact like Farcaster. Imagine lots of agents with wallets and budgets transacting in a digital economy.
This framework is no doubt oversimplified. The advantaged approaches in each quadrant have opportunities to win across scenarios. Primarily, products that enable new behavior, create new forms of access, and inspire users to adopt them will create outsized value. But these quadrants have helped our team think through “what has to happen for this approach to be most advantaged?” in a moment of particularly outsized unknowns.
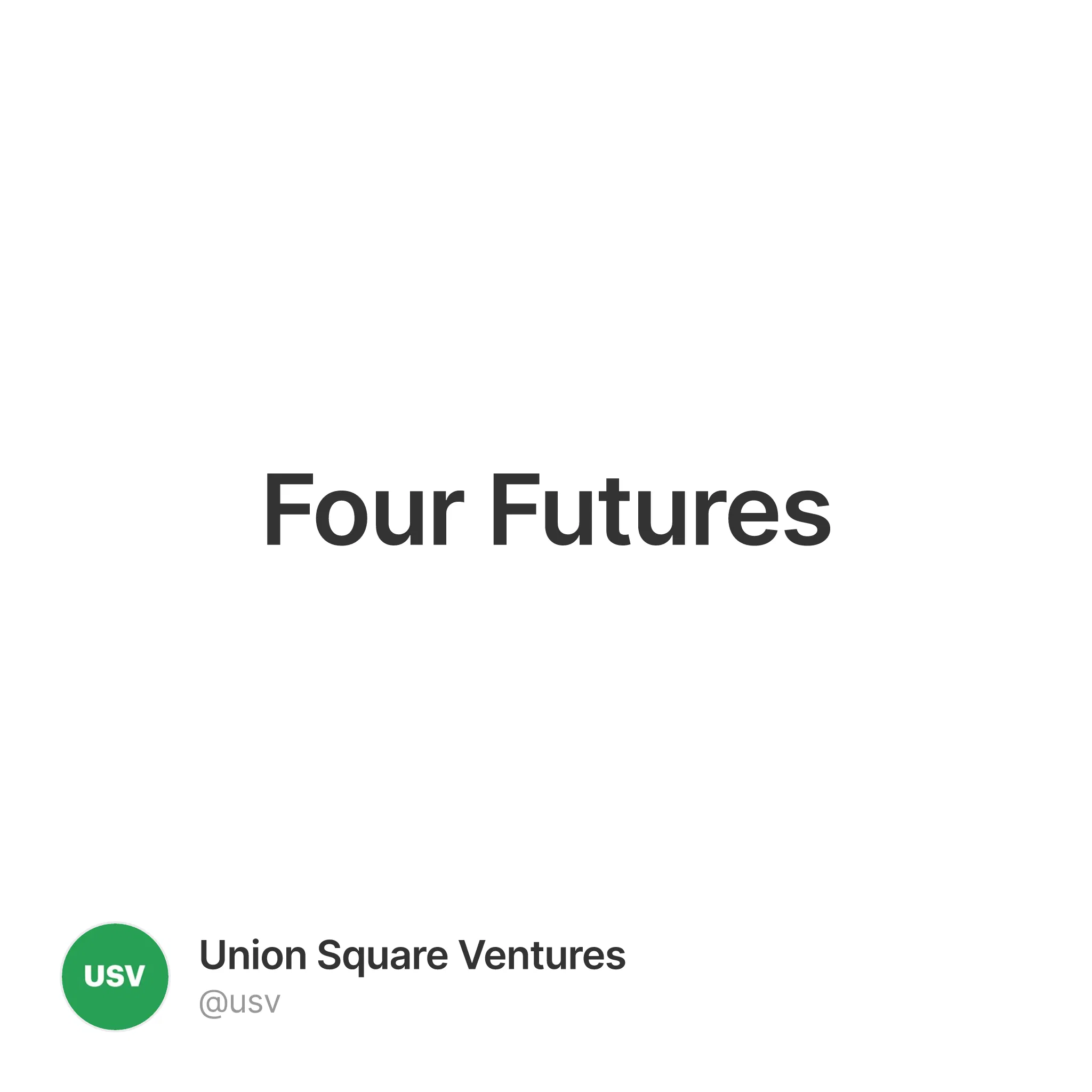
